Generative Artificial Intelligence (Gen AI) and especially Large Language Models (LLMs) have captured the attention of the media and a society that is asking questions and discussing the impact of the technology. But amidst all this noise and current hype, there are relevant opportunities for companies, regardless of their size, and they must adapt their time and resources to take advantage of this technological disruption.
In brief, LLMs (like OpenAI’s GPT-3.5 or GPT-4, but there are much more) are pre-trained models (also called foundation models) based on transformers deep learning architectures like OpenAI’s GTP-3.5 or GPT-4, the basis of the famous chatbot ChatGPT. But even though they are general models that can serve any purpose (multidomain and multitask), there is not a one-size-fits-all solution, especially for commercial enterprises looking to adopt Gen AI in their unique, data-driven use cases. For all the positives that Gen AI services can bring to businesses, they do not do so without their own adaptation, risks, and downsides.
In any case, what is certain is that LLMs have the potential to transform almost any industry and we’re only at the dawn of this new Gen AI Era. From Telefonica Open Innovation we are in touch with the most innovative generative AI startups. Don’t get left behind and learn how they can help you design your business strategy in generative AI.
Understanding Gen AI
Let’s start from the beginning: An Artificial Intelligence Model is a type of software that aims to replicate and amplify intelligent human behaviour, with minimal human intervention. Models in general are basically a representation of real-world objects or behaviours. AI models in particular are a representation of intelligent processes, currently inspired by human intelligence.
The big difference between the latest generation of Gen AI and the more established types of AI (analytic, discriminative) is that it leaps from cognitive capabilities (and outputs) into the realm of creative capabilities (and outputs). The computer outputs new (original) information rather than simply recognizing or classifying existing content.
Gen AI is therefore a subset of AI that can create realistic images, videos, music, fashion, design, code, materials, synthetic data and can accelerate the rate of scientific progress, to name just a few applications of the tech.
After the successful launch of ChatGPT in November 2022 by OpenAI and its partnership with Microsoft, all MAGMA companies have multiplied their efforts in Gen AI and LLMs in particular. Apart from the plans to integrate the technology in their products and services, with Gen AI Microsoft, Google, and Amazon leverage their cloud infrastructure business. Regarding ChatGPT, in addition to the model open to the B2C by OpenAI (and retrained by humans every day), Microsoft is pioneering the space starting to offer closed instances for enterprises, to work directly with OpenAI’s APIs and to achieve more controlled and secure results. But this is not the only option. Open source LLMs and the new trend small LLMs are gaining traction and we still don’t know what shape the final landscape of Gen AI solutions for businesses will take.
Gen AI has an impact on the workforce
Gen AI impacts businesses at three levels. Firstly, it transforms the profile and skills of the workforce, that can multiply their productivity. Gen AI enhances the productivity of knowledge workers by automating repetitive tasks and homogenising and improving the quality of work. According to OpenAI data, around 80% of the US workforce will experience at least 10% task disruption and 19% faces a more substantial 50% impact.
The main challenge is determining whether technology will replace or overshadow human workers. However, the prevailing view is that AI is meant to augment human capabilities rather than replace them. To overcome this challenge, reskilling and upskilling the workforce are crucial. By focusing on roles that require human skills and creativity, knowledge workers can thrive alongside Gen AI technologies.
Impact on the company
Secondly, the adoption of Gen AI by the industry is expected to be massive, with some sectors being early adopters and others following suit. Internal use of Gen AI has a significant impact on providing efficiencies for companies.
However, the widespread adoption of Gen AI also presents challenges. One challenge is the need for expertise in AI to fully understand and leverage the potential of this technology. The fragmented and specialized nature of the technology offerings in this field can make it difficult for companies to find suitable solutions that fit their specific needs. The lack of expertise may lead to a potential increase of operational costs.
Another significant challenge is to minimise the risk of hallucinations, the potential misuse, and the associated ethical concerns. Gen AI can be used to create deepfakes, spread disinformation, commit financial fraud, and exploit biometric authentication, among other risks. Companies must proactively address these ethical and security concerns to mitigate the potential risks associated with the misuse of generative AI.
Impact on end products and services
And lastly, Gen AI unlocks new functionalities for existing products and the creation of products that were previously unimaginable. The ability of machines to generate original content offers companies new avenues for innovation and market differentiation.
In brief, Gen AI applicability extends to any software or application that utilizes text, images, voice, or video. This includes graphic design, content creation, music production, automatic translation, dialogue generation, and more.
In terms of trying to understand the effects that Gen AI generates on companies´ products and services, the first statement that must be done is that Gen AI is not the product, or at least not the end product itself but rather a piece of technology used to enhance existing products and services or create new ones. It serves as an additional component that enables companies to deliver more personalized and creative experiences.
When integrating Gen AI, by fine-tunning pre-trained models can save time and money. The availability of pre-trained models in Gen AI offers companies an efficient way to implement this technology in their products and services. These pre-trained models are developed by specialised companies and contain self-learned or semi-supervised knowledge and patterns from large datasets. By utilizing them, companies can save time and resources that would otherwise be spent on training models from scratch, thus expediting the implementation process and reducing associated costs.
Building the Gen AI strategic plan for your company
There are several factors to consider when adopting generative AI for the enterprise. On one hand, the capabilities that Gen AI brings to products follow the pace set by the maturity of the foundational models that enable them. As time goes on, the models will be able to respond more accurately to expert vertical contexts, and the synergies with traditional analytical AI and automation will increase, unlocking their full potential.
In this context, McKinsey’s three temporal horizons can serve as a reference. This framework encompasses different aspects of strategic planning and development in three stages for companies to navigate different timeframes and develop a comprehensive strategy that balances short-term gains, medium-term growth, and long-term resilience in the face of disruptive change.
- Horizon 1 (H1) focuses on efficiencies and productivity improvements in the near term. It involves identifying and implementing measures to optimize existing processes, reduce costs, and enhance operational effectiveness. H1 initiatives often involve streamlining workflows, automating repetitive tasks, and leveraging technologies to drive efficiency gains and maximize productivity. Most of the cross-industry use cases proposed in this article are H1.
- Horizon 2 (H2) revolves around exploring new services and business opportunities that can be pursued in the medium term. This horizon involves identifying emerging trends, market gaps, and customer needs to develop innovative products or services. H2 initiatives focus on diversifying offerings, expanding into new markets, and establishing competitive advantages through differentiation and value creation.
- Finally, Horizon 3 (H3), the horizon of disruption, is concerned with long-term transformation and the potential impact of disruptive technologies or business models. It involves anticipating and preparing for significant industry shifts, disruptive innovations, and changing customer behaviours. H3 initiatives entail exploring radical new ideas, fostering experimentation, and proactively adapting the business to stay ahead of disruptive forces.
Some Gen AI cross-industry use cases
There are many use cases of generative AI for enterprises that are already a reality, although their implementation is not always straightforward. Setting up the necessary infrastructure to enable them, integrating with the company’s legacy systems, and, in some cases, the need for upskilling the workforce, make their adoption not as fast and smooth as desired. The use cases presented hereafter are our selected quick-wins:
- Employees knowledge base: In this field we can find the startup unicorn Glean, an AI-powered enterprise search and knowledge discovery solution for teams to search and pull together information from all company apps, documents, conversations, tickets and more.
- Marketing and Sales assistant: Brand content generation, hyper-personalization of communication (from B2C to B2Me), user profiling or creating new business proposals based on context. Two unicorns are taking everyone’s attention: Jasper, an AI-marketing creator to make fast, easy, original and creative content for your blog, social media, website, and more. Likewise, Synthesia allows to generate AI videos from plain text in minutes.
- Virtual assistants and Call Center: Customized virtual avatars, improved understanding, automatic transcription, conversation analysis, summarization and characterization, intelligent routing, data classification and structuring, sentiment and veracity detection. In this line, Character.ai is another Gen AI startup that has hit the unicorn status, their value proposition is based on allowing their customers to create their own personalized AI chatbot using LLMs and deep-learning algorithms.
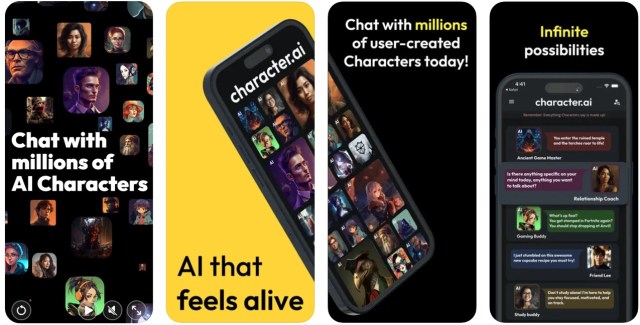
- Software development: Model encoding, code explanation and commenting, automatic code generation, error prevention and QA testing tools. There are a lot of examples of startups hitting this field, GitHub Copilot, and AI pair programmer (and of course, a unicorn) is an intelligence AI tool that turns natural language propmts into coding suggestion across dozens of languages. Other companies such as Tabnine are also offering an AI assistant for software developers.
- Data: Generation of synthetic data, data classification, pattern identification, natural language data analytics and explanation of data in natural language. Here, Mostly.AI is an AI-powered synthetic data generator of high-quality and privacy-safe synthetic versions of datasets for ML, advanced analytics, software testing and data sharing.
- Legal, Financial and Transparency: Automatic report generation, contract and taxes specifications generation. Seed companies like DraftWise and Originality.ai provide solutions for documents generation and AI generated content detection respectively.
About investment in Gen AI startups
In the current economic situation, characterized by high interest rates, military conflicts, and bankruptcies of major banks that traditionally financed startups, there has been a decline in Venture Capital funding. However, in this adverse scenario, the primary startups successfully raising capital are those based on AI. According to Pitchbook, Venture Capitalists have increased their investment in Gen AI by 425% since 2020, reaching $2.1B by beginning of January 2023. A significant portion of this capital is likely being used to develop foundation models, platforms, and the necessary infrastructure.
From the start of January 2023, Gen AI companies have managed to secure a total of $14.1 billion in equity funding through 91 deals, which includes the significant investment of $10 billion made by Microsoft in OpenAI. Even without considering the OpenAI transaction, this represents a noteworthy 38% growth compared to the total funding obtained throughout the entirety of 2022.
Opportunities for startups
The first wave of Gen AI applications are built by startups on top of foundation models and are starting to reach scale, but struggle with retention, differentiation and gross margins.
The Gen AI applications space is growing rapidly: three product categories have already exceeded $100 million of annualized revenue: copywriting, coding and image generation.
The success stories in the three categories above emphasize the need of human expertise to do a good job (the well-known examples of Jasper, assisting and not replacing the human copywriter, GitHub Copilot, a pair programmer that assists coding or Midjourney a bot assistant that produces images based on natural language description uniquely and artistically).
The next promising Gen AI category seems to be conversational AI: specialized chatbots (ChatGPT-like) like those provided by Liveperson, using LLMs, fine-tuned for specific purposes and guaranteeing privacy and security may disrupt business of traditional chatbots that do not use generative AI.
Regarding the business model, current solutions offer gross margins of 50-60% due to high cost of hosting and processing (20% and 20% aprox. respectively). These margins should improve as competition and efficiency in foundation models increases.
Still some big questions remain around how applications built on top of foundation models will compete with vertically integrated solutions or how startups will develop their go to market strategy, building features for existing solutions (to ease go-to-market) or creating new standalone apps (competing to attract customers).
In our view, there seems to be an unmet opportunity for startups building Composite AI products (combining generative + analytic capabilities) for B2B customers requiring specialised solutions beyond LLM services provided by big players.